Today, Pew Research Center released the first major report from its Data Labs team, examining the degree to which partisan conflict shows itself in congressional communications – specifically, press releases and Facebook posts. We sat down with Solomon Messing, who directs Data Labs, to discuss the report, the project’s mission and the opportunities and challenges that come with using “big data.” The conversation has been edited for space and clarity.
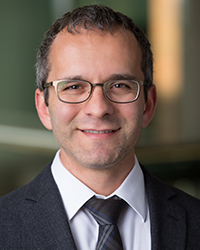
First off, what does Data Labs do?
We use approaches from the emerging field of what I would call “computational social science” to complement and expand on the Center’s existing research agenda. What we do generally is collect text data, network data or behavioral data and analyze it with new and innovative computational techniques and empirical strategies.
So this goes beyond the Center’s traditional emphasis on public opinion surveys?
Surveys are a fantastic way to study a wide swath of social science questions. The reason to expand into these new areas might be to study things that you can’t get at using survey data – because you can’t survey a particular group of people, or because you need fine-grained evidence about what people do, which they may be unable or unwilling to report accurately in surveys.
In the context of this report, for example, it’s really difficult to study the substance of congressional rhetoric in a survey: We would have had a hard time getting every member of Congress to take a survey, and even if they did they might have trouble reporting exactly how often they “go negative” in their public outreach.
Another key point here is that these approaches allow us to supplement survey data with additional types of data. People might not know the average income in their ZIP code, but using that data can add another dimension to what we know about public opinion.
Give some examples of the kinds of data you work with.
Well, in this first report we collected text data – press releases and social media posts from members of Congress – and used a combination of human coders and machine learning to analyze it. That lets us look for patterns, like the relationship between how often members criticize the other side in public outreach and the partisan composition of people in their district.
We also collect network data – data that describe the connections between people or things. For example, we’re looking at how people share URLs on social media. That can be represented as a network of people sharing common URLs, which then can be used in a number of interesting ways, such as identifying groups of people with common interests or identifying URLs that are aligned with a liberal or conservative audience.
So you’re using these sorts of “big data” techniques to study what people do, as opposed to what they say they do?
Exactly – it’s about studying the digital trails people leave behind, rather than what they tell us on surveys. Using these approaches to study social science questions has only recently become possible. Thanks largely to the proliferation and declining cost of digital storage, much more of the information in the world can be captured and stored as data. That means we can now access or collect or create data that we couldn’t previously get. And we also now have the resources to acquire and analyze these datasets that we just didn’t have before, thanks to cheaper computing infrastructure, readily deployable tools and a robust community of people dedicated to advancing these approaches who share insights and code online.
So, instead of surveying people about what we’re interested in, your research involves getting answers from data that people generated in the course of doing something else in their everyday lives?
That’s right, which means we have to be really careful about what we ask of the data. And another note of caution: Because this kind of data is tremendously complex, it’s a little easier to mistake noise for signal if you don’t take the proper safeguards. If I look at every single word in every single statement from every single member of Congress, I’m going to find a lot of words that are associated with, say, taking a vote on a particular bill that are just there by chance. This is a very common problem when analyzing very complex datasets – if I’m examining thousands of different variables, I’m bound to find associations just by chance. So we need to design research projects carefully and guard against finding spurious relationships.
Earlier you used the phrase “machine learning.” Can you explain what that is?
Generally it refers to using computer algorithms to learn from data without explicit instructions from a human. In most cases that’s done by using statistical models to build up associations based on models of inputs and outputs, which then are used to make data-driven inferences, often that characterize some body of data.
Let’s say we have a series of social media posts and we want to teach a machine to learn whether or not those posts are discussing politics. If we have a few thousand posts that we already know discuss politics, we can feed those to the computer, and it can build up a model of the kinds of words and phrases that are used in political posts versus nonpolitical posts. Then we can use that model to take a new post and infer whether or not it is in fact discussing politics. Machine learning is particularly helpful when the dataset you want to analyze is very large: If we’re looking at 200,000 posts, it would take a human far too long to actually read every single one.
Why did you decide to study congressional communication as Data Labs’ launch project?
It helps us understand a key link between lawmakers and the pubic they represent. These communications illustrate how Congress is expressing its priorities and exercising leadership. And what people say to their representatives is one of the few connections they have to them, outside of voting. But because this kind of work is so difficult to execute, there’s less of it than in the realm of public opinion more broadly.
What made you decide to focus specifically on press releases and Facebook posts? Were there any other sources of data you considered using, such as tweets, transcripts of talk-show appearances, or floor speeches printed in the Congressional Record?
Press releases are a very explicit, official statement of policy to the public, and because they’re often covered in the media we can use them to better understand the dynamic between Congress, the media and the public. Facebook posts are another form of communication that members use to communicate with the public – obviously a much newer form of communication, but it’s becoming increasingly prominent. Nearly every member of Congress now has an official Facebook account.
We’re also very interested to see how, or if, the patterns we saw in the 114th Congress change with the new Congress and the new administration.
In the course of pulling this project together, were there any unexpected roadblocks or challenges that you encountered?
There were a lot of challenges to overcome, but one stands out to me as something folks in this field need to pay careful attention to, and that is the issue of machine-learning bias.
One thing we wanted to measure was when a post was attacking the other side – a Democrat attacking a Republican or vice versa. In the 114th Congress, Republicans criticized Obama more often than Democrats criticized Republicans – most likely because at the time they were the presidential “out party.” Now, if we blindly apply our machine-learning models to every instance of criticism, what will happen is that the computer will better learn the word patterns that Republicans use in criticizing the other side than Democratic patterns, simply because it has more Republican examples to train on. Left unchecked, that means the machine will have a harder time detecting critical rhetoric from Democrats, and that would exaggerate the differences between the two sides.
Instead, what we decided to do was oversample critical rhetoric from Democrats, and build separate machine classifiers for Democrats and Republicans. That gave the machine classifier enough examples of various criticisms from Democrats that in the end, it performed about as well for both parties.
In the course of doing this project, what did you learn about the capabilities and limitations of machine learning?
One thing we learned that it’s incredibly difficult to train a computer to recognize something as subtle and sensitive as “indignant disagreement.” Part of it is that it’s incredibly difficult to train a human to do that, because it’s essentially such a subjective problem. So it took us a number of iterations to get it right.
With the humans or with the machines?
Both.
Was there any particular type of text that the computer had special difficulty with?
The data that we collected from press releases was from hundreds of different websites, and each one of those websites is formatted slightly differently. Some members include boilerplate text in their press releases, and if you’re not careful that can really confound your analysis. One example we found is that some members note in every one of their press releases that they’re on some bipartisan committee, and the word “bipartisan,” even just encountering it once, can sometimes fool the machine into thinking that the entire content of the release is meant to be bipartisan. So we built a system to detect and remove that kind of boilerplate before we asked the machine to classify each text.
What findings do you consider particularly noteworthy or surprising?
I was a little bit surprised to find that members of Congress tended to express more disagreement in their press releases than in their Facebook posts. Also, engagement was much higher on critical Facebook posts than on other posts. Finally, it turns out that Republicans tend to favor Facebook – they post more often on Facebook than they release press releases, whereas Democrats tend to favor the traditional route. That was a little counterintuitive to me because in the past decade Democrats developed a reputation for using digital technology and social media to wage effective campaigns.
I imagine many laypeople might think of big data as a way to overcome the kinds of error and imprecision inherent in things like survey research. But your report talks about how the big-data approach contains its own sources of error.
It’s tremendously important that people understand that. There are a number of different sources of error that characterize any attempt at data analysis, whether it’s survey research or computational social science.
First of all, things have to be conceptualized properly in terms of research design. That’s particularly important in this new field, where the data being used was not produced to answer the questions you might be asking of it. There’s error in operationalization of different concepts, just as there is in survey research. There’s error when I tell a computer to try to measure some kind of sentiment in the data. There’s noise when it comes to aggregating responses – for example, aggregating a collection of machine-learned texts for one member of Congress – which is similar in some ways to sampling error. And finally there’s measurement error – the machine-learning system can just misclassify something.
Has the field gotten to the point where there are norms for what is considered an acceptable error rate – the equivalent of the 95% confidence level in survey research, for example?
I think we’re still developing those norms, but often it depends on the question. One thing that many people in the machine-learning field think a lot about is what’s an acceptable misclassification rate, and is it better to have false positives or false negatives? That’s a balance you have to strike. It might be that we care a lot about making sure we don’t miss any instances in which a member is saying something that is bipartisan; then we want to do everything we can to minimize the false-negative rate. Or it might be that we care about the opposite – we really don’t want to say that a member is being indignant when they’re not – in which case we care a lot about the false-positive rate. So it’s really important to think through which kind of error is worse. Those are more important questions than “Have we reached an accuracy rate above 90 percent?”
What lessons can you take from this project and apply to future projects?
I think the first lesson is that it’s really important to get a good sense of what data you have, what you need, and what kinds of supplementary data provide the biggest bang for the buck. In this project, we had to obtain, process and validate a bunch of unstructured text data that no one else had put together, and that took us a lot of time and effort. But after we did that, we were able to bring in data sources that other researchers already had put together, such as an ideology measure for individual members of Congress. By building directly on that existing work, we were able to map out some important implications of the findings in our original data analysis.
Relying on commonly used data standards is really important, because it will minimize any work you need to do to implement some other algorithm that someone might have published online. For example, one commonly used standard is JSON [JavaScript Object Notation, a language-independent data format], when you’re collecting data or sending it from one system to another. There are standard ways of parsing it, and it has a lot of features designed to make it easily accessible. It makes the 90 percent of the time that’s put into these projects on data processing far less onerous.
And I think piloting as much as possible on small subsets of the data is really important. That prevents you from sinking a lot of effort into wrangling a really big data source and then finding out after the computer’s been running for a week that actually it’s not going to work, that you did something wrong.
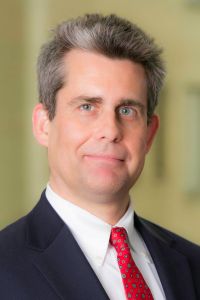