The analysis in this report is based on respondents to a set of self-administered web surveys conducted between December 2015 and March 2018. The analysis is restricted to those individuals who were originally recruited from two large telephone surveys in 2014 and 2015 to join Pew Research Center’s American Trends Panel (ATP), and remained active panelists through at least July 2017.
The ATP is a nationally representative panel of randomly selected U.S. adults recruited from landline and cellphone random-digit-dial surveys. Panelists participate via monthly self-administered web surveys. Panelists who do not have internet access are provided with a tablet and wireless internet connection. During the first several waves, the panel was managed by Abt, and it is currently being managed by GfK.
Of the more than 8,000 respondents who were recruited to the ATP in 2014 and 2015, 7,588 were asked questions in their recruitment surveys about support for the tea party (in the 2015 survey, a random ¾ of respondents were asked this question). This analysis is based on the 3,974 who remained active panelists through at least 2017 and restricted to the 1,724 who identified with or leaned to the Republican Party in the December 2015 survey.
The following table shows the unweighted sample sizes and the error attributable to sampling that would be expected at the 95% level of confidence for different groups in the analysis:
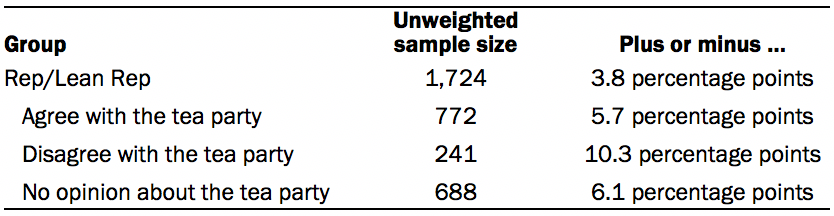
Sample sizes and sampling errors for other subgroups are available upon request.
In addition to sampling error, one should bear in mind that question wording and practical difficulties in conducting surveys can introduce error or bias into the findings of opinion polls.
About the missing data imputation
Participants in the American Trends Panel are sent surveys to complete roughly monthly. While wave-level response rates are relatively high, not every individual in the panel participates in every survey. The analysis of Republican tea party supporters is restricted to respondents who completed the summer 2017 “member engagement” survey (a yearly survey to update information about panelists). Data in this analysis includes questions asked on waves conducted December 2015; April, September and November 2016; and February and March 2018.
Several hundred respondents did not respond to at least one of these waves. A statistical procedure called multiple imputation by chained equations was used to guard against the analysis being undermined by this wave level nonresponse. There is some evidence that those who are most likely to participate consistently in the panel are more interested and knowledgeable about politics than those who only periodically respond. Omitting the individuals who did not participate in every wave of the survey might introduce bias into the sample.
The missing data imputation algorithm we used is a method known as multiple imputation by chained equations, or MICE. The MICE algorithm is designed for situations where there are several variables with missing data that need to be imputed at the same time. MICE takes the full survey dataset and iteratively fills in missing data for each question using a statistical model that more closely approximates the overall distribution with each iteration. The process is repeated many times until the distribution of imputed data no longer changes. Although many kinds of statistical models can be used with MICE, this project used classification and regression trees (CART). For more details on the MICE algorithm and the use of CART for imputation, see:
Azur, Melissa J., Elizabeth A. Stuart, Constantine Frangakis, and Philip J. Leaf. March 2011. “Multiple Imputation by Chained Equations: What Is It and How Does It Work.” International Journal of Methods in Psychiatric Research.
Burgette, Lane F., and Jerome P. Reiter. Nov. 1, 2010. “Multiple Imputation for Missing Data via Sequential Regression Trees.” American Journal of Epidemiology.